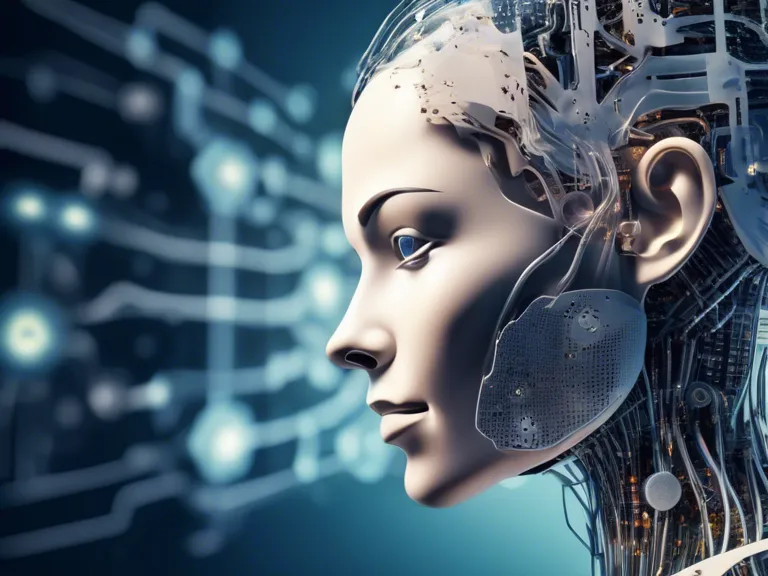
Introduction
Machine learning has revolutionized various industries by enabling machines to learn from data and make decisions without explicit programming. With recent advancements in technology and the availability of vast amounts of data, the field of machine learning is progressing rapidly. In this article, we will explore how to accelerate progress in machine learning to achieve rapid gains.
Leveraging Data for Training
Data is the fuel that powers machine learning algorithms. To accelerate progress in machine learning, organizations need to focus on collecting high-quality data and ensuring it is labeled accurately. By leveraging diverse and well-annotated data sets, machine learning models can be trained more effectively, leading to better performance and faster learning.
Utilizing Advanced Algorithms
Advancements in machine learning algorithms, such as deep learning and reinforcement learning, have significantly improved the capabilities of machine learning models. By utilizing these advanced algorithms, organizations can achieve rapid gains in performance and accuracy. It is crucial to stay updated with the latest research and developments in the field to leverage the most cutting-edge algorithms for your applications.
Embracing Automation and Scalability
Automation and scalability are key factors in accelerating progress in machine learning. By automating the process of model training, hyperparameter tuning, and deployment, organizations can save time and resources while achieving faster results. Additionally, leveraging scalable infrastructure, such as cloud computing services, allows for efficient training of models on large data sets, leading to rapid gains in performance.
Continuous Learning and Adaptation
Machine learning models need to continuously learn and adapt to new data and changing environments to maintain their effectiveness. By implementing strategies such as online learning and reinforcement learning, organizations can ensure that their models are constantly improving and evolving. Continuous learning and adaptation are essential for achieving rapid gains in machine learning.
Collaborating and Sharing Knowledge
Collaboration and knowledge sharing within the machine learning community are crucial for accelerating progress in the field. By participating in conferences, workshops, and online forums, practitioners can exchange ideas, collaborate on projects, and learn from each other's experiences. Sharing knowledge and best practices can help drive innovation and lead to rapid gains in machine learning.
Conclusion
Accelerating progress in machine learning for rapid gains requires a combination of leveraging data effectively, utilizing advanced algorithms, embracing automation and scalability, promoting continuous learning and adaptation, and fostering collaboration and knowledge sharing. By following these strategies and staying updated with the latest trends and developments in the field, organizations can achieve significant advancements in machine learning and drive innovation across various industries.
acme-nuclear.com
agilityimap.com
akikcombong.com
anniesmysteries.com
bflofoodie.com
brandzonestudios.com
cacemar.com
daperezlaw.com
denvyautomation.com
eugeneband.com
factory-eshop.com
florentdumas.com
hishaywireless.com
in2-signs.com
kuwekeza-holdings.com
mitaniya-ltd.com
mixfoure.com
mobilitypluspro2.com
moipravila.com
montreal-business-kit.com
mortiseandmiter.com
nextdigitaldental.com
nurdalilahputri.com
oem-phoneaccessories.com
palmbeachestepona.com
precavida.com
roscoeandetta.com
scriptsnmacros.com
sringserver.com
thecustomfairy.com
withlovefromangela.com
applebyandwood.com
auzigog.com
eac-w.com
homesbyelevation.com
nihilismforoptimists.com
slavonkandhortus.com
thekoreanpolitics.com
turningpointpt.com
val-up.com
wakansen.com
3dideation.com
achilles-fire.com
banatelhalal.com
biyografirehberi.com
bohams.com
comisiondeestudios.com
cooride-net.com
danayumul.com
ecadecom.com
edwardscornerfarmersmarket.com
ekspresif.com
ellajmae.com
ginroecooks.com
gracefueled.com
hightidekitchen.com
jeroenswolfs.com
marthalott.com
mollybroekman.com
mpthoidai.com
plumfashionpr.com
racktents.com
solzapower.com
southcoastbehavioralhealth.com
the101bali.com
thearguide.com
theartistfia.com
thefitnesswire.com
thelivelihoodproject.com
thelynndentonagency.com
wilkespoolsnspas.com
wjwatson.com
drinkganbei.com
mendenhallnews.com
nathaliemoliavko-visotzky.com
nationalinfertilityday.com
wide-aware.com
ashleymodernfurniture.com
babylonbusinessfinance.com
charliedewhirst.com
christianandmilitaryhats.com
hypnosisoneonone.com
icelandcomedyfilmfestival.com
kayelam.com
mlroadhouse.com
mumpreneursonline.com
posciesa.com
pursweets-and.com
rgparchive.com
therenegadehealthshow.com
travelingbitz.com
yutakaokada.com
22fps.com
aarondgraham.com
essentialaustin.com
femdotdot.com
harborcheese.com
innovar-env.com
mercicongo.com
oabphoto.com
pmptestprep.com
rmreflectivevest-jp.com
tempistico.com
filmintelligence.org
artisticbrit.com
avataracademyagency.com
blackteaworld.com
healthprosinrecovery.com
iancswanson.com
multiversecorpscomics.com
warrenindiana.com
growthremote.com
horizonbarcelona.com
iosdevcampcolorado.com
knoticalpr.com
kotaden.com
la-scuderia.com
nidoderatones.com
noexcuses5k.com
nolongerhome.com
oxfordcounselingcenter.com
phytacol.com
pizzaropizza.com
spotlightbd.com
tenbags.com
thetravellingwilbennetts.com
archwayintl.com
jyorganictea.com
newdadsplaybook.com
noahlemas.com
qatohost.com
redredphoto.com
rooms4nhs.com
seadragonenergy.com
spagzblox.com
toboer.com
aumantvmuseum.com
beyondausten.com
citylabstudio.com
diskonio.com
drinkcf.com
eft-dongle.com
emilymeganphotography.com
evolveathleticclub.com
godleystationvet.com
hirochanweb.com
homeonefurniture.com
ifiwasastylist.com
lacantinepopup.com
liriklagubatak.com
lo-ko.com
mensagenseatividades.com
myway-zeus.com
nevadadec.com
nokarikhabar.com
nuuuki.com
quenchpad.com
sckyrock.com
tindunghanoi.com
tradeshows-biz.com
wikimuzik.com