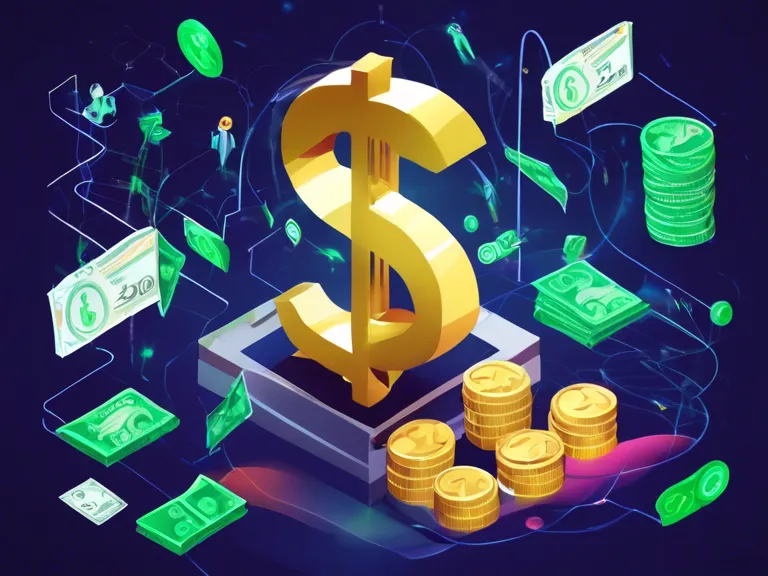
Introduction
Machine learning has revolutionized various industries, including finance, by providing powerful tools for data analysis, prediction, and decision-making. In the realm of financial forecasting, machine learning algorithms have proven to be instrumental in leveraging vast amounts of data to make accurate predictions about future market trends and investment opportunities.
Importance of Financial Forecasting
Financial forecasting plays a crucial role in the realm of finance as it helps businesses, investors, and financial institutions make informed decisions about investments, risk management, and strategic planning. By using historical data, market trends, and other relevant information, financial forecasting enables stakeholders to anticipate future outcomes and mitigate potential risks.
Machine Learning in Financial Forecasting
Machine learning algorithms excel in handling large volumes of data and identifying complex patterns that may not be apparent to human analysts. In financial forecasting, machine learning models can analyze historical market data, economic indicators, news sentiment, and other factors to make accurate predictions about stock prices, market trends, and asset values.
One of the key advantages of using machine learning in financial forecasting is its ability to adapt and learn from new data, allowing the models to continuously improve their predictive accuracy over time. This adaptability is particularly valuable in the dynamic and ever-changing landscape of financial markets.
Common Machine Learning Techniques in Financial Forecasting
1. Time Series Analysis
Time series analysis is a fundamental technique in financial forecasting that involves analyzing historical data to identify patterns and trends over time. Machine learning models such as ARIMA (AutoRegressive Integrated Moving Average) and LSTM (Long Short-Term Memory) are commonly used to analyze time series data and make predictions about future values.
2. Regression Analysis
Regression analysis is another widely used technique in financial forecasting that involves predicting a continuous value based on the relationship between independent variables. Machine learning algorithms such as linear regression, support vector machines (SVM), and random forest regression are commonly used for regression analysis in financial forecasting.
3. Neural Networks
Neural networks are a class of machine learning models inspired by the structure and function of the human brain. In financial forecasting, deep learning neural networks, such as convolutional neural networks (CNN) and recurrent neural networks (RNN), are used to analyze complex data patterns and make accurate predictions about market trends and asset prices.
Challenges and Limitations
While machine learning has shown great promise in financial forecasting, there are several challenges and limitations to consider. One of the main challenges is the potential for overfitting, where a model performs well on training data but fails to generalize to new, unseen data. Additionally, interpreting the decisions made by machine learning models can be challenging, especially in complex financial scenarios where the factors influencing outcomes are not easily discernible.
Conclusion
Machine learning has significantly enhanced the capabilities of financial forecasting by enabling the analysis of vast amounts of data and identifying complex patterns that can inform investment decisions and risk management strategies. By leveraging machine learning techniques such as time series analysis, regression analysis, and neural networks, financial institutions and investors can gain valuable insights into market trends and make more informed decisions. As technology continues to advance, the integration of machine learning in financial forecasting is poised to reshape the future of finance and investment strategies.