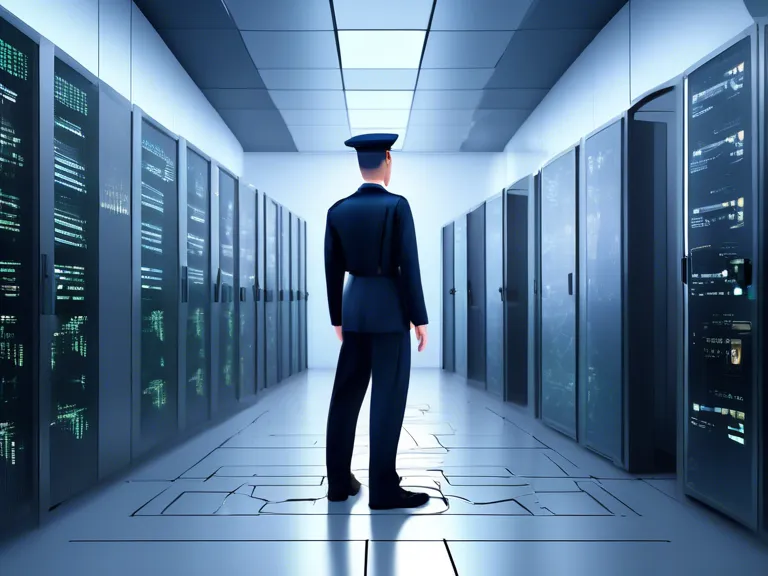
Introduction
Data machine learning applications have revolutionized security guard systems by providing advanced capabilities in threat detection, surveillance, and decision-making. However, these applications are also vulnerable to various security risks and attacks. In this article, we will explore the importance of defending data machine learning applications in security guard systems to ensure their effectiveness and integrity.
The Role of Machine Learning in Security Guard Systems
Machine learning algorithms play a crucial role in enhancing the capabilities of security guard systems. These algorithms can analyze vast amounts of data, detect patterns, and make informed decisions in real-time. By leveraging machine learning, security guard systems can identify potential threats, predict security breaches, and optimize response strategies.
Security Risks in Data Machine Learning Applications
While data machine learning applications offer significant benefits, they are susceptible to various security risks. Adversaries can exploit vulnerabilities in machine learning models to manipulate results, launch targeted attacks, or compromise the integrity of the system. Common security risks in data machine learning applications include data poisoning, model inversion attacks, and adversarial examples.
Defending Data Machine Learning Applications in Security Guard Systems
To safeguard data machine learning applications in security guard systems, organizations need to implement robust defense mechanisms. Here are some key strategies for defending data machine learning applications:
Data Security
Ensuring the security and integrity of training data is essential for the reliability of machine learning models. Organizations should implement encryption techniques, access controls, and data validation processes to protect sensitive data from unauthorized access and tampering.
Model Monitoring
Continuous monitoring of machine learning models is crucial to detect anomalies, deviations, or potential attacks. Organizations should establish monitoring systems that track model performance, inputs, and outputs to identify any suspicious activities or changes in behavior.
Adversarial Training
Adversarial training involves augmenting machine learning models with adversarial samples to improve their robustness against attacks. By exposing models to adversarial examples during training, organizations can enhance their resilience and ability to withstand malicious inputs.
Regular Updates and Patches
Keeping data machine learning applications up to date with the latest security patches and updates is essential to mitigate vulnerabilities and protect against emerging threats. Organizations should establish a proactive approach to software maintenance and ensure timely deployment of security fixes.
Threat Intelligence Sharing
Collaborating with industry peers and sharing threat intelligence can help organizations stay informed about potential security risks and attack vectors. By participating in information sharing initiatives and leveraging collective knowledge, organizations can enhance their defense strategies and mitigate evolving threats.
Conclusion
Defending data machine learning applications in security guard systems is imperative to ensure the reliability, integrity, and effectiveness of these systems. By implementing robust security measures, organizations can mitigate security risks, protect sensitive data, and enhance the resilience of their machine learning models. It is essential for organizations to stay vigilant, proactive, and adaptive in their approach to defending data machine learning applications in security guard systems.